Ilya Sutskever's SSI: Inside the $32B Quest for Safe Superintelligence (2025)
What's Ilya been cookin with Safe Super Intelligence?
No AI media hype cycle is complete without intermittently discussing the clandestine operation that is SSI (Safe Superintelligence Inc.), a company founded by the rarefied AI talent that is Ilya Sutskever. (Random: AutismCapital pointed out the fact that SSI is eerily similar to SSRI… just add an R and you’re a class of serotonergic antidepressants.)
In the world of elite AI talent, Ilya is a considered the crème de la crème.
Elon Musk co-founded OpenAI along with Sam Altman and Greg Brockman in 2015. Elon recruited a lot of the elite talent, including Ilya, noting that Ilya was one of his “toughest hiring battles.”
Technically Ilya Sutskever is also regarded as a co-founder of OpenAI (after being recruited by Musk) and served as OAI’s Chief Scientist, playing a major role in the organization’s AI research.
In November 2023, Sutskever was among the OpenAI board members who voted to oust Sam Altman as CEO, a decision driven by concerns that OpenAI was moving too quickly on powerful AI without sufficient safety measures.
Ilya would later backtrack, expressing regret for his vote/decision to axe Altman and publicly supported Altman’s reinstatement as CEO.
This led many AI hype fiends to constantly spam the question “What did Ilya see?” (you still see this on X/Twitter occasionally in 2025).
Anyways, Ilya lost his OpenAI board seat and maintained a low public profile until his departure in May 2024. When Ilya left OpenAI, many were saying “OpenAI is cooked” sans Ilya… clearly this wasn’t the case.
Elon Musk attempted to poach Sutskever to xAI (Grok), but Sutskever declined and instead quickly formed his own company SSI (Safe Superintelligence Inc.) in June 2024 with partners Daniel Gross and Daniel Levy with the aim of developing “safe superintelligence” free from commercial pressures.
The company operates with notable secrecy — its website is “bare bones,” employees seldom disclose their affiliation, and strict confidentiality measures are in place.
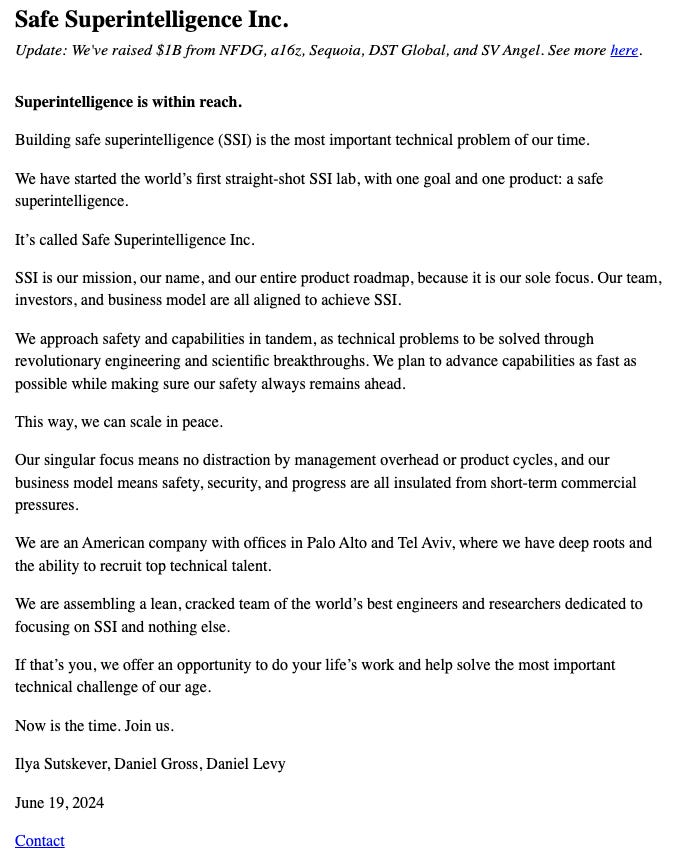
What’s so impressive about Ilya Sutskever? His reputation stems from his foundational contributions to deep learning, including:
AlexNet (2012): Co-author of the groundbreaking paper that popularized convolutional neural networks for image recognition.
Sequence-to-Sequence Learning (2014): Helped pioneer a powerful neural approach for machine translation, which underpins many modern language models.
Generative Models (GPT series): Played a key part in the development of OpenAI’s GPT models, critical to the transformer revolution in natural language processing.
Deep Reinforcement Learning: Contributed to notable DRL successes, such as OpenAI’s Dota 2 bot.
AI Safety and Superalignment: Shifted his focus at OpenAI toward controlling superintelligent AI, co-leading its “Superalignment” team.
Many perceive Ilya Sutskever as a real-world manifestation of Nathan Bateman, the guy from the movie Ex Machina living in an isolated luxurious home who builds an ultra-smart humanoid robot named Ava with AGI/ASI. (They obviously differ in certain ways: Ilya has high moral/ethical standards — the covertness and genius are similar.)
Collaborating with o3, I wanted to speculate on various aspects of what SSI is actually doing… will they actually be competitive with major AI companies like OpenAI, Anthropic, Google, Meta, DeepSeek, Qwen, et al.? Or is Ilya et al. too far behind to have any shot at catching the lead?
I.) Safe Superintelligence Inc: 2025 Overview
Safe Superintelligence Inc. (SSI) is a highly-funded artificial intelligence startup founded in mid-2024 by Ilya Sutskever (formerly OpenAI’s chief scientist), alongside Daniel Gross and Daniel Levy.
The company’s singular mission is to achieve a safe superintelligence—an AI system that exceeds human intelligence while remaining aligned with human values.
Unlike product-driven AI labs, SSI has adopted a distinct “straight-shot” approach, focusing exclusively on long-term artificial general intelligence (AGI) research rather than short-term commercial applications.
This intentional, focused strategy sets SSI apart from other players in the AI space.
As of April 2025, SSI has rapidly emerged as one of the most valuable AI startups, despite having no public-facing product or demo.
Its rise is attributed to the prestige of its founding team and substantial backing from major investors, including leading tech giants.
Founders:
Ilya Sutskever – Former Chief Scientist at OpenAI
Daniel Gross – AI investor and entrepreneur
Daniel Levy – Former key researcher at OpenAI
Mission: To build a safe superintelligence, prioritizing alignment and safety at every stage of development.
Strategy:
Pure research focus on AGI
No short-term commercial distractions
Streamlined organizational structure to minimize bureaucracy and maximize scientific output
Funding & Valuation:
Raised a total of $3 billion as of early 2025
Valued at $32 billion
Backed by prominent tech investors including Alphabet and Nvidia
Product Status:
No public product, API, or demo
Full focus on internal AGI development and safety frameworks
Positioning: SSI is uniquely positioned in the AGI race as a deep-research, safety-first organization. It stands apart from AI labs balancing research with enterprise use cases, aiming instead for a singular, high-stakes breakthrough.
II.) SSI: Team & Talent (April 2025)
Size & Composition: SSI remains extremely lean, with roughly 20 employees globally as of early 2025. The team is split between Palo Alto, California and Tel Aviv, Israel, reflecting the co-founders’ roots in both Silicon Valley and the Israeli tech ecosystem. This small headcount is striking given the company’s multibillion-dollar valuation, underscoring SSI’s strategy of concentrating top talent rather than building a large workforce.
Founders & Key Talent: Beyond Sutskever, who serves as Chief Scientist, SSI’s other co-founders bring notable credentials:
Daniel Gross – Chief Executive Officer (CEO): A former Apple AI lead and Y Combinator partner, Gross oversees fundraising and compute strategy. He is responsible for ensuring the startup has the massive computing power needed for frontier AI research and has been a key liaison with investors and cloud providers. Gross has emphasized building a “small highly trusted team” and spends extensive time vetting candidates for extraordinary ability and good character.
Daniel Levy – Principal Scientist: An ex-OpenAI researcher, Levy is a respected AI scientist co-leading SSI’s research efforts. His background at OpenAI gives SSI additional experience in large-scale model development and safety research. Levy’s role under Sutskever is to drive the technical research agenda toward safe superintelligent models.
In addition to the founding trio, SSI has started to attract elite researchers:
Dr. Yair Carmon – Senior Research Engineer: One of SSI’s first hires in Tel Aviv, Dr. Carmon is a machine learning expert who joined from academia. A Stanford PhD and Tel Aviv University lecturer, his research focuses on robust and reliable ML algorithms. Carmon’s recruitment signals SSI’s ability to draw top academic talent; he will help build out the Tel Aviv R&D presence.
Other Talent: While most hires remain undisclosed, SSI’s “lean, crack team” likely includes individuals with strong backgrounds in AI research and engineering (e.g. ex-Google Brain, DeepMind, or OpenAI engineers). Gross noted they prioritize “people with extraordinary capabilities” over those with just impressive resumes. By April 2025, the team was still growing, especially in the new Tel Aviv office, but SSI has kept a low profile on specific personnel beyond the co-founders and a few key recruits.
Talent Strategy: SSI’s approach to talent is to hire slowly and selectively to ensure alignment with the company’s culture and mission. Trustworthiness and a long-term mindset are emphasized, given the sensitive goal of developing safe AGI. This contrasts with larger labs that scaled up rapidly; SSI is intentionally “small and highly trusted”. The presence of an office in Israel (where Sutskever grew up) also gives SSI access to Israel’s strong pool of AI researchers and engineers. Overall, SSI’s team – though tiny – includes world-class AI minds and is expected to expand moderately as needed to tackle its ambitious R&D roadmap.
III.) Funding & Strategic Backing of SSI
Despite its short existence, SSI has secured extraordinary funding from top-tier investors, reflecting strong confidence in its mission and leadership:
Initial Funding (Mid-2024)
In September 2024, just three months after its launch, SSI raised $1 billion in cash at a valuation of approximately $5 billion.
This unprecedented seed round was led by premier Silicon Valley venture firms including Andreessen Horowitz (a16z), Sequoia Capital, DST Global, and SV Angel (via Nat Friedman).
The size and speed of this raise—particularly for a company with no product—highlight the degree to which investors were willing to make outsized bets on exceptional talent.
Much of the funding hinged on Ilya Sutskever’s reputation as the scientific mind behind many of OpenAI’s major breakthroughs.
Insiders described the investment as a hedge in the AI race, anchored in belief that Sutskever could replicate or surpass his earlier successes at a new lab purpose-built for safety-focused AGI.
Series A / Growth Funding (Early 2025)
As of April 2025, SSI closed a second round of funding worth approximately $2 billion, bringing its valuation to $32 billion. This 6-fold increase in less than a year reflected dramatic investor enthusiasm.
This round was led by Greenoaks Capital, which contributed a $500 million check. Returning investors like a16z, Lightspeed Venture Partners, and DST Global also participated. In a notable shift, strategic tech giants joined this round: Alphabet (Google’s parent) and Nvidia both took equity stakes in SSI.
Alphabet’s involvement is partly strategic, as Google Cloud was selected as SSI’s TPU provider.
Nvidia’s stake aligns it with one of the most ambitious next-gen AI labs, potentially giving it insight into and influence over future AI infrastructure demands.
Although exact dollar amounts from Alphabet and Nvidia were not disclosed, their backing added significant credibility and validation to SSI's efforts from two of the biggest players in the AI ecosystem.
Investor Motivations
Several key drivers have fueled SSI’s ability to attract capital at scale:
Betting on Elite AI Talent: Investors are fundamentally betting on Ilya Sutskever—a rare figure who helped lead projects like AlexNet, GPT, and DALL·E. His role in OpenAI’s rise has earned him deep trust among investors, who now see SSI as a potential challenger to OpenAI, Anthropic, and Google DeepMind. SSI’s top-tier talent roster enhances the likelihood that it could produce another foundational model to reshape the AI landscape.
Hedge Against Other AI Bets: For companies like Alphabet, investing in SSI acts as a hedge—ensuring they retain exposure to external AGI breakthroughs. This mirrors strategies used with other labs, such as Anthropic. VCs, too, may view SSI as a “second chance” to back a generational AI company after missing early stakes in OpenAI or Anthropic.
Belief in the Mission (Safety Focus): SSI’s explicit focus on AI safety and alignment distinguishes it from many competitors and appeals to investors wary of unchecked AGI development. Backers like Nat Friedman are known for supporting high-risk, long-term tech ventures rooted in positive societal outcomes, making SSI an attractive fit.
Hype & Mystique: There is a clear element of mystique surrounding SSI. With no product, no public demos, and a “stealth” brand, the company has cultivated a sense of FOMO (fear of missing out) among investors. The drama around Sutskever’s departure from OpenAI only intensified interest, and the rapid escalation in valuation has reinforced the perception that SSI could be the next great leap in AI. Some analysts have called the valuation “staggering,” but note that in the rarefied world of frontier AI, the rules of conventional valuation often don’t apply.
Scarcity of Top AI Talent: At the frontier of AI development, talent is scarce. Very few individuals and teams are capable of building safe, superintelligent systems. SSI’s funding is therefore not just a bet on a company—it’s a bet on access to one of a very limited number of credible AGI efforts in existence. Investors are effectively buying into compute access, algorithmic advancement, and top-tier researchers.
Use of Funds
SSI’s leadership has made it clear that its capital will go primarily toward:
Compute Infrastructure: Access to cutting-edge chips and supercomputers for model training
Elite Talent Acquisition: Competitive compensation for world-class AI researchers and engineers
Unlike most startups, SSI is not spending on user acquisition, marketing, or even product development in the short term. Instead, its entire budget is dedicated to long-term R&D.
CEO Daniel Gross emphasized that SSI aims to spend “a couple of years [on] R&D” before considering market entry—buffered from short-term commercial pressures thanks to its deep capital reserves.
Gross further remarked that “raising capital is not going to be one of [their problems],” signaling strong confidence in continued investor support as long as scientific progress is maintained.
SSI’s funding history illustrates a rare convergence of visionary leadership, elite investors, strategic tech partners, and bold long-term thinking. Despite having no public product, the startup has raised $3 billion and reached a $32 billion valuation within its first year—an astonishing feat even in the hyper-accelerated AI sector.
Investors are clearly driven by a mix of belief in Sutskever’s ability to lead the next AI breakthrough, a desire to own a piece of a scarce AGI opportunity, and the pragmatic calculus of not being left behind in the most important technological race of the century. With significant capital, a mission-driven culture, and a high-trust team, SSI is positioned to be a defining force in the future of superintelligent AI.
IV.) Compute Infrastructure (2025)
To build superintelligent AI, Safe Superintelligence Inc. (SSI) requires compute at frontier scale—and from day one, they’ve made that a priority.
Despite its small team, SSI commands a disproportionate share of computing resources due to deep capital reserves and strategic alignment with the world’s most powerful cloud infrastructure.
🔌 GPU/TPU Resources
SSI is reported to be an anchor customer for Google Cloud’s AI supercomputers. In April 2025, Alphabet’s cloud division struck a deal to provide SSI with large-scale access to Tensor Processing Units (TPUs)—one of the largest external TPU commitments Google has made to date.
Google’s custom AI chips, not Nvidia GPUs, are SSI’s primary compute engine.
Google reportedly agreed to sell SSI chips in “significant quantities” to support its frontier AI research.
Though exact numbers aren’t public, “significant” implies tens of thousands of TPU cores or more, comparable to the infrastructure behind models like GPT-4.
This makes SSI one of the only independent labs operating at true supercomputing scale — but entirely through cloud-based infrastructure, not physical datacenter buildouts.
🧠 Strategic Compute Alignment
SSI’s compute strategy is explicit:
Secure massive TPU allocations up front to remove scaling constraints.
Conduct massive training runs remotely, leveraging Google’s data centers.
Focus entirely on research, while Google handles physical scaling and maintenance.
In its initial $1B funding round, SSI earmarked capital specifically to “acquire computing power.” CEO Daniel Gross has played a direct role in this, overseeing relationships with Google and ensuring infrastructure readiness.
This relationship is mutually beneficial:
Google gains a marquee customer demonstrating TPU superiority.
SSI receives cutting-edge chips, scalable infrastructure, and potentially early access to new hardware like TPU v5.
💠 Nvidia’s Position
While TPUs dominate SSI’s architecture, Nvidia is not excluded:
Nvidia is an investor in SSI.
SSI may maintain a small GPU cluster for compatibility with CUDA-based tools, legacy experiments, or redundancy.
The investment ensures future flexibility — should GPUs ever become a better fit.
That said, at core scale, SSI has clearly bet on TPUs. This separates them from most peers, including OpenAI, Meta, and academic labs, who rely primarily on Nvidia GPUs. SSI aligns more with Anthropic and DeepMind, who also deploy extensively on TPU infrastructure.
🖥️ Supercomputing Scale
All indicators suggest SSI is operating at a GPT-4-class or higher compute scale:
The deal likely involves multiple TPU pods, networked together into a virtual “supercluster.”
With $3 billion raised, SSI can afford multiple large training runs, each costing tens to hundreds of millions in cloud compute.
Their target—to develop a model that "far surpasses human capabilities"—implies a need for compute 10× greater than GPT-4, by some estimates.
This scale is not just theoretical. SSI’s arrangement likely includes:
TPU v5 access, possibly including early or experimental versions.
Deployment in Google Cloud's major AI infrastructure regions—Iowa, Oregon, or similar.
No signs of a private SSI datacenter yet, meaning cloud-first remains the operating model.
🧑💻 Infrastructure Team
Though unpublicized, SSI must operate with a core group of elite systems engineers:
Massive distributed training—across thousands of TPUs—requires expertise in parallelism, sharding, networking, and fault tolerance.
Team likely includes ex-Google, DeepMind, or OpenAI infrastructure talent.
Cloud-native workflows allow SSI to scale with fewer people by outsourcing ops to Google, allowing researchers to focus solely on model development.
Google Cloud’s startup partnership director summarized the trend:
“With these foundational model builders, the gravity is increasing dramatically over to us.”
SSI is at the forefront of that shift.
🧾 Compute Philosophy: Spend on Scale
From a first-principles perspective, SSI’s entire compute posture is purpose-built:
Buy scale instead of building it slowly.
Trade capex for opex—leasing power instead of constructing it.
Front-load compute access to eliminate the classic AI bottleneck: not enough hardware.
Where OpenAI and others ramped up over years, SSI entered the race already fully fueled. This gives them an enormous advantage in iteration speed, experiment scope, and technical ambition.
SSI has quietly assembled one of the most powerful AI compute stacks in the world.
Primary infrastructure: Google TPUs at massive scale, including likely access to TPU v5 and future chip generations.
Secondary capacity: Some GPU presence (or potential future expansion via Nvidia), maintaining ecosystem flexibility.
Training capacity: Enough to support hundreds of billions of parameters and multiple frontier-scale runs.
Operational model: Fully cloud-native, lean team, rapid iteration.
This makes compute a non-issue for SSI—an intentional move. As Sutskever and Gross see it, the real challenge isn’t chips; it’s what you do with them.
Their supercomputing pipeline is already live. It’s the engine behind their “straight-shot” to safe superintelligence. With hardware fully secured, SSI’s fate now depends on one thing: whether their algorithms and alignment strategy can deliver on the mission.
V.) Progress & Roadmap
As of early 2025, Safe Superintelligence Inc. (SSI) remains deep in its research and development phase, with no public product and a deliberately long runway before any release. The company’s focus is singular: building safe superintelligence—not shipping early demos or minimal viable products.
🗓️ Operational Timeline
Founded: June 2024, immediately following Ilya Sutskever’s departure from OpenAI.
First 6–10 months: Spent in setup mode—securing funding, hiring core staff, and beginning infrastructure and research buildout.
As of April 2025: No published papers, demos, or public benchmarks. SSI remains in stealth mode by design.
Rather than incremental rollouts, SSI is pursuing a “go slow to go fast” philosophy—similar to DeepMind’s early strategy. There is no chatbot, no API, and no technical documentation. The company is focused entirely on long-term AGI development without commercial distraction.
🔬 Internal R&D Focus
SSI’s first year appears centered around building foundational capabilities for future model development. Likely steps include:
Infrastructure buildout (largely completed by end of 2024, enabled by Google Cloud TPU partnership).
Training early models (e.g., 10B–50B parameters) to test alignment strategies and system behavior before scaling up.
Iterative safety testing, experimenting with reward models, internal agent simulations, and constraint enforcement.
Full-scale training, expected sometime in 2025 or 2026, likely involving a 500B+ parameter model using their custom compute stack.
Gross has stated they intend to spend “a couple of years on R&D” before thinking about commercialization, reinforcing that their roadmap is long-term and safety-first.
🚫 No Public Demos (Yet)
As of early 2025, SSI has not released any product, model, or research paper.
This is intentional: SSI is focused on developing a highly capable and aligned system before public exposure.
Internal milestones likely exist (e.g., achieving performance thresholds, alignment benchmarks), but have not been shared.
Their decision to remain quiet is strategic, avoiding the risk of hype cycles or rushed releases. The approach mirrors early DeepMind, which revealed breakthroughs only when they were validated and meaningful (e.g., AlphaGo).
🎯 Stated Goals & Milestones
Though technical details are scarce, SSI’s mission implies ambitious targets:
“AI systems that far surpass human capabilities” across domains.
Solve or mitigate the alignment problem—ensuring safety scales with intelligence.
Build a true AGI—a generally capable, adaptive system—not just another narrow LLM.
Do all of this safely, under tight internal controls before any external exposure.
Sutskever has publicly stated he believes superintelligence could arrive this decade, and SSI is structured to lead that effort—while avoiding premature deployment.
🧩 Potential Product Strategy
SSI has suggested that the system itself is the product. When (and if) it is deemed safe, several deployment pathways are possible:
API Access or Cloud Platform. Similar to OpenAI’s model delivery, but likely more restricted. Access could be tightly controlled and safety-gated for select partners or institutions.
Enterprise or Scientific Solutions. Use in domains like research, climate modeling, or bioengineering—where aligned, high-intelligence systems could offer massive value under strict oversight.
Internal Use for AI Safety. Possibly using early AGI prototypes to help evaluate or align even more advanced models—a concept known as recursive alignment assistance.
Phased Introduction. Controlled rollouts, perhaps starting with private evaluations, problem-solving demonstrations, or supervised assistant use cases. This would build public and institutional trust before broader release.
Gross has emphasized the desire to “scale in peace”, avoiding short-term market pressure. The product rollout will reflect this philosophy.
📅 Timeline Expectations
2024–2025: Infrastructure setup, early experiments, hiring top researchers, securing funding.
2025–2026: Train SSI’s first large-scale model (possibly 500B+ parameters), test alignment mechanisms, and refine capabilities.
Late 2026–2027: Potential private evaluations, cautious unveiling, and phased deployment of the superintelligent system.
Beyond: Use AGI to support alignment research, partner with scientific or global institutions, or accelerate safe AI governance.
⚠️ Caveat: This timeline is speculative and could shift based on research success or technical hurdles. AGI is not guaranteed on a schedule.
🔍 Indirect Signals of Progress
While no technical results are public, several external signals suggest momentum:
Talent Attraction: The hiring of researchers like Dr. Yair Carmon and steady team growth (~20 staff as of early 2025) point to credible internal progress. Top-tier talent wouldn’t join a failing lab.
Investor Confidence: The $2B Series A at a $32B valuation in early 2025 suggests insiders saw strong internal metrics, plans, or model results. Even without public proof, internal benchmarks likely impressed major investors like Alphabet and Nvidia.
No Leaks, No Stalls: There have been no reports of delays, staff exits, or failed experiments. The narrative around SSI remains disciplined and optimistic.
🔭 Outlook: What Comes Next
The next 1–2 years will be critical:
If successful, SSI may quietly train and evaluate an AGI-class model by late 2025 or early 2026.
If difficulties arise, timelines may stretch—but no public indicators currently suggest this.
Key inflection point will be internal safety validation—SSI likely won’t move forward until they can verify the alignment of their system.
SSI’s roadmap is long, focused, and capital-rich. The company is:
Avoiding early product cycles
Spending years on R&D without revenue
Building a single transformative system—not incremental tools
Their strategy is to achieve safe superintelligence first, and only then decide how to share it with the world. Every sign—from funding to hiring to communication style—suggests they are methodically executing on that plan.
In the AGI race, SSI isn’t sprinting for headlines—they’re building toward a controlled finish line.
VI.) SSI Model Architecture & Approach
As of April 2025, Safe Superintelligence Inc. (SSI) has not released any public AI model or technical demonstration.
The company has remained deliberately opaque about its architectural choices.
However, a combination of the team’s background, public statements, and hardware decisions allows for informed speculation about SSI’s direction.
Transformer-Based Foundation
It is widely assumed that SSI’s foundational model builds on the transformer architecture—the same paradigm that powers the most advanced large language models (LLMs) today.
Ilya Sutskever co-authored the original 2017 Transformer paper and led the development of GPT-3 and GPT-4 at OpenAI. Given this history, it is highly likely that SSI is continuing with this architecture as a proven base.
There is no indication that SSI has introduced an entirely new architecture. Rather, their efforts seem focused on scaling known architectures to new levels while embedding safety from the ground up.
Their mission to advance “capabilities… while making sure our safety always remains ahead” strongly suggests a parallel development track for performance and safety features.
Safety & Alignment Focus
What may set SSI apart is its deep focus on AI alignment and safety as core architectural concerns, not afterthoughts.
Sutskever’s previous work on OpenAI’s “Superalignment” team likely informs SSI’s approach. From the beginning, SSI may be incorporating architectural features such as:
Interpretability tools to monitor internal reasoning
Robust reward models for grounded behavior
Advanced constraints or monitoring to ensure alignment
Rather than relying solely on Reinforcement Learning from Human Feedback (RLHF)—the dominant technique used by others—SSI may explore new safety-first design principles as part of its core research.
The company has publicly stated: “We approach safety and capabilities in tandem, as technical problems to be solved through revolutionary engineering.”
Use of Google TPUs
A confirmed architectural choice is SSI’s use of Google Cloud’s Tensor Processing Units (TPUs) instead of Nvidia GPUs—a rare move among independent AI labs.
TPUs, especially the v4 and v5 chips, are highly optimized for dense matrix operations and large-scale neural network training.
TPUs typically align with JAX and TensorFlow, suggesting SSI’s software stack may be optimized for Google’s tools and research frameworks.
This shift might enable faster iteration cycles or more scalable training efficiency, and possibly even close collaboration with Google researchers on optimization.
While not a direct architectural change, TPUs could influence architectural decisions by favoring designs that leverage their strengths.
This choice positions SSI outside the mainstream (which leans heavily on Nvidia), potentially giving them distinct performance insights or cost advantages as they scale.
Hypothetical Comparison to Leading Architectures
Without public details on SSI’s architecture, we can hypothesize its approach by comparing it to leading AI models, focusing on their training methodologies, safety mechanisms, and reasoning capabilities.
Below, we analyze how SSI might align with or diverge from key players, grounding speculation in industry standards and SSI’s safety-first ethos.
OpenAI’s GPT and o-Series: OpenAI’s GPT-4o is a transformer-based, multimodal large language model (LLM) trained on vast datasets, fine-tuned with Reinforcement Learning from Human Feedback (RLHF), and capable of text, vision, and audio processing. Its o-series (o1, o3-mini, o3) marks a leap in reasoning, using test-time computation and process supervision to enable chain-of-thought reasoning, achieving top benchmarks (e.g., 87.7% on GPQA). OpenAI applies RLHF post-pretraining for GPT models and integrates safety checks during inference for o-series, balancing capability and alignment. SSI likely employs transformer-based LLMs, given their dominance and Sutskever’s OpenAI background, but may prioritize intrinsic safety during pretraining—potentially modifying loss functions or embedding alignment constraints—unlike OpenAI’s post-training alignment. SSI’s scale and multimodal ambitions are unknown, but its AGI focus suggests it aims to match or exceed o-series reasoning and safety, possibly via novel training protocols. Without disclosures, these parallels remain speculative.
DeepSeek’s R1, R1-Zero, and V3: DeepSeek’s R1, R1-Zero, and V3 are open-weight, transformer-based large language models (LLMs) that combine supervised pretraining on large corpora with reinforcement learning (RL) fine-tuning, such as process supervision, to enhance reasoning. R1 rivals OpenAI’s o1 in math and coding benchmarks, while R1-Zero, a lightweight variant, offers similar reasoning at reduced computational cost, broadening accessibility. V3 matches GPT-4o’s performance at a training cost of $5.58 million, leveraging efficient data curation and RL. Claims of DeepSeek using “pure” RL without pretraining are inaccurate; all three models rely on hybrid training for stability and performance. SSI may draw inspiration from DeepSeek’s cost-efficient RL techniques or R1-Zero’s lightweight design to optimize reasoning and safety, but its proprietary focus and AGI goals suggest custom architectures over adopting open-weight models. A plausible SSI approach is hybrid training—pretraining for broad knowledge followed by RL to embed safety and reasoning—aligned with industry standards but tailored to its “safety ahead of capabilities” mission. DeepSeek’s open-source contributions, such as training methodologies and evaluation frameworks, could indirectly inform SSI’s benchmarking or data strategies.
Google’s Gemini 2.0 & DeepResearch: Google’s Gemini 2.0 Flash and DeepResearch models are transformer-based, multimodal LLMs supporting text, vision, and tool use, with reasoning enhanced by search integration and APIs. They improve on Gemini 1.0/1.5, which lagged behind GPT-4, but still trail o1 and Claude 3.5 in some benchmarks. Claims of leveraging DeepMind’s AlphaGo heritage are unverified, as these models rely on transformers, not RL systems like AlphaGo’s Monte Carlo Tree Search. Google’s agentic features (e.g., memory, multi-step reasoning) are application-driven, not core architectural shifts. SSI, backed by Alphabet’s TPUs, may explore reasoning or agentic capabilities for AGI, but its “different mountain” (per Sutskever’s 2024 comment) likely emphasizes intrinsic safety over Google’s product-oriented AI (e.g., Search, Assistant). SSI’s architecture remains undisclosed, but it may prioritize foundational safety mechanisms-agents over application-specific features, differing from Google’s ecosystem focus.
Anthropic’s Claude: Anthropic’s Claude Sonnet, a transformer-based LLM, uses Constitutional AI—self-supervised RL aligning outputs with a “constitution” of ethical principles—to prioritize safety and reduce human feedback compared to RLHF. It rivals GPT-4o and o1 in reasoning and coding, setting a high safety benchmark. SSI shares Anthropic’s safety-first ethos and may explore advanced alignment, such as intrinsic safety mechanisms, AI feedback loops, or adversarial training, potentially integrating these during pretraining rather than Anthropic’s fine-tuning focus. Without technical disclosures, SSI’s alignment methods are speculative, but its AGI ambitions suggest it aims to surpass Claude’s safety and reasoning, possibly via novel self-critique or value-alignment protocols. The two differ in scope: Anthropic targets safe, deployable AI, while SSI pursues foundational AGI.
xAI’s Grok: xAI’s Grok 3, a proprietary 10-trillion-parameter transformer-based LLM, is trained from scratch on a 200,000 Nvidia H100 GPU cluster, not built on open-source models as previously claimed. Optimized for truth-seeking and conversational clarity, Grok 3 competes with o1 and Claude 3.5 in reasoning (e.g., 85% on GPQA) and supports xAI’s scientific discovery goals. Unlike SSI’s pre-product focus, Grok is deployed for X Premium+ users, balancing rapid release with AGI ambitions. SSI likely trains from scratch for AGI, emphasizing safety over early deployment, but both share a frontier-level scope. SSI may diverge by embedding safety deeper into training, contrasting xAI’s post-training fine-tuning for conversational and ethical alignment. Grok’s open-sourced Grok 1 code could inform SSI’s benchmarking, but SSI’s proprietary approach prioritizes leapfrogging existing models.
Open-Source Models (LLaMA, Qwen, DeepSeek): Open-weight models like Meta’s LLaMA (research-only), Alibaba’s Qwen, and DeepSeek’s R1/V3 offer strong performance at smaller scales, with DeepSeek rivaling GPT-4o and o1. SSI is unlikely to adopt these models, given its proprietary focus and goal to “leapfrog the state of the art.” However, SSI may leverage open research methodologies—such as chain-of-thought prompting, efficient data curation, or evaluation frameworks (e.g., DeepSeek’s training insights)—to enhance reasoning, safety, or benchmarking. Open-source models set a competitive baseline, but SSI’s $3 billion funding and Alphabet/Nvidia backing suggest a custom, resource-intensive approach to AGI, distinct from community-driven efforts.
While SSI’s exact architecture remains undisclosed, all indicators suggest that it is building a massive transformer-based language model, likely augmented with novel safety, alignment, and interpretability features.
The use of Google TPUs, coupled with a commitment to from-scratch model development, positions SSI to potentially diverge from the mainstream in important ways.
What sets SSI apart may not be what they build, but how they build it:
Embedding safety and alignment from the beginning
Pursuing innovative training pipelines
Leveraging alternative hardware ecosystems
And applying scientific discipline rather than commercial urgency
If successful, SSI’s architecture could not only rival current frontier models but redefine what it means for an AI system to be both superintelligent and safe.
Radical New Paradigm for SSI Architecture?
At face value, SSI’s approach does not yet appear to be a radical new paradigm (they are not openly touting a brand-new architecture different from transformers).
Instead, the novelty may lie in how they combine and scale existing ideas.
My analysis of what’s needed for superintelligence suggests a few avenues SSI might explore for differentiation:
Scale: SSI likely pursues large-scale training to achieve advanced capabilities, a strategy shared by OpenAI (o-series, 87.7% GPQA) and xAI (Grok 3, 10T parameters). With $3 billion and access to Alphabet’s TPUs, SSI can target frontier-level models, potentially matching or exceeding Grok 3’s scale or DeepSeek’s V3 ($5.58M training, GPT-4o parity). By focusing on a single model, SSI may allocate compute more efficiently than diversified labs like Google, which juggles Search and Assistant projects. However, competitors like xAI (200,000 H100 GPUs) and OpenAI are already pushing hardware limits, so SSI’s scale is unlikely to be uniquely novel unless it surpasses these by a significant margin (e.g., tens of trillions of parameters), which current hardware constraints make challenging. The hypothesis that extreme scale could trigger an AGI emergent leap—yielding qualitatively new capabilities like Nobel laureate-level performance (per Dario Amodei’s AGI definition, March 6, 2025)—is plausible but debated. Scaling enhances reasoning (e.g., o3’s benchmarks) but faces diminishing returns, as we discussed on February 20, 2025, suggesting SSI may need architectural innovation or novel data paradigms to achieve AGI.
Reinforcement Learning & Agency: SSI may integrate RL more prominently to develop general problem-solving abilities, aligning with its “revolutionary engineering” ethos. RL techniques, such as process supervision in DeepSeek’s R1/R1-Zero (rivals o1 in math/coding) or test-time computation in OpenAI’s o-series, enhance reasoning and could enable SSI’s model to achieve goals in simulated environments or dialogue. Unlike Google’s Gemini 2.0, which uses application-driven agentic features (e.g., tool use, search integration) rather than deep RL, SSI might explore RL to foster autonomous multi-step reasoning or self-improvement, critical for AGI. For example, training a model to refine its own reasoning could be a breakthrough. However, no public evidence confirms SSI’s RL approach, and such advancements remain speculative. SSI’s focus on AGI suggests it builds on transformer-based LLMs, potentially using hybrid training (pretraining + RL fine-tuning) like DeepSeek, but tailored to safety and generality.
Alignment by Design: SSI’s most distinctive potential lies in architecting models with safety embedded from the ground up, diverging from OpenAI’s post-training alignment (e.g., RLHF for GPT-4o, inference-time safety for o3). Sutskever’s departure from OpenAI suggests differing views on safety, and SSI’s mission implies intrinsic alignment strategies, such as training on safety-filtered datasets, using modular architectures for auditability, or developing novel sandboxing to limit unintended behaviors. These could resemble Anthropic’s Constitutional AI, but integrated earlier, potentially during pretraining via modified loss functions or AI feedback loops. A general solution to controlling superintelligent models—SSI’s stated goal—would require conceptual breakthroughs, possibly drawing from control theory or formal verification, but no public details outline SSI’s methods. While speculative, such an alignment-centric architecture would distinguish SSI from GPT-style models and set a new safety benchmark, forcing competitors to adopt similar innovations if successful. For now, SSI likely trains a large, transformer-based model with enhanced safety mechanisms, aiming for a reliably aligned system capable of surpassing human intelligence in a controlled manner.
Outlook: SSI’s success hinges on delivering architectural or training innovations that surpass models like o3, Claude 3.5, and Grok 3. Its $3 billion funding and single-model focus provide a strong foundation, but its pre-product status and the competitive landscape (e.g., DeepSeek’s cost-efficiency, xAI’s scale) pose challenges. If SSI achieves a breakthrough in safe AGI, it could redefine industry standards. Until technical disclosures emerge, SSI’s approach remains a transformer-based LLM with safety-first enhancements, pursuing AGI through scale, RL, and alignment innovation.
VII.) Advanced Speculation on SSI Architectures
Included below is more detailed speculation on the potential architectures SSI may be using for its AI.
This is based off of all known information as of April 2025. Understand that information may rapidly shift such that architectures and probabilities estimated in this section become obsolete.
Keep reading with a 7-day free trial
Subscribe to ASAP Drew to keep reading this post and get 7 days of free access to the full post archives.